Greener Future Through Vision AI and Ultralytics YOLO
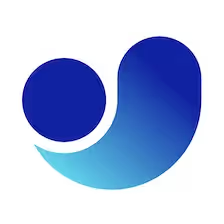
October 10, 2023
Discover TrashBestie, an innovative app using Ultralytics YOLOv8 for smarter waste sorting with AI. Join the eco-friendly movement with a digital solution.

October 10, 2023
Discover TrashBestie, an innovative app using Ultralytics YOLOv8 for smarter waste sorting with AI. Join the eco-friendly movement with a digital solution.
TrashBestie is a new app that helps us sort and manage waste in a different and better way using computer vision. TrashBestie uses deep learning and advanced technology to help people take action to make the planet cleaner and more sustainable.
The team behind TrashBestie envisions a future where waste is no longer a nuisance but an opportunity for positive change. Sorting waste is important for protecting the environment, saving resources, and reducing pollution. With this in mind, TrashBestie became the digital solution that empowers individuals to make informed waste management decisions effortlessly. The goal is clear: inspire a collective movement toward responsible waste management and foster a cleaner planet for generations to come.
Before we dive into the innovative technology behind TrashBestie, let's meet its creators:
Helge began studying machine learning during his master's thesis, examining how a manager's pay relates to a company's success. This involved using regression models and machine learning techniques. Helge was able to dive deeper into the world of vision AI at Spiced Academy's Bootcamp. Here, he experimented with deep learning and determined the usefulness of Ultralytics YOLO models.
My had a friend who shared his data science projects, which sparked her interest in machine learning. The way data could uncover insights and optimize processes fascinated her. That's why she joined the Bootcamp, where she met Simantini and Helge.
Simantini started exploring machine learning during her master's thesis. She discovered its potential in her field of work, which involves assessing building damages caused by earthquakes. Following her graduation, Simanti had different jobs involving data. These jobs eventually led her to a data science bootcamp and piqued her interest in ML and vision AI.
TrashBestie's use of Ultralytics YOLOv8 as the primary tool is strategic.
TrashBestie operates as a personal waste-sorting assistant, using artificial intelligence to simplify the process into four straightforward steps:
The development journey of TrashBestie involves a series of crucial steps:
TrashBestie is continuing to improve by adding localization, making it more accessible on iOS and Android, and refining image processing techniques. The team is committed to continually improving the app's performance and precision.
Check out their project on Devpost, which includes an image gallery and a YouTube video showcasing the details of their work.
TrashBestie is on a mission to revolutionize waste management and make our planet cleaner and more sustainable. This is a first step into the future, that could even revolutionize the conception of waste management careers. Join them on this exciting journey toward a greener future!