Explore Stefano Puliti's transformative forest research using YOLOv5 for drone-based detection and analytics in forestry.
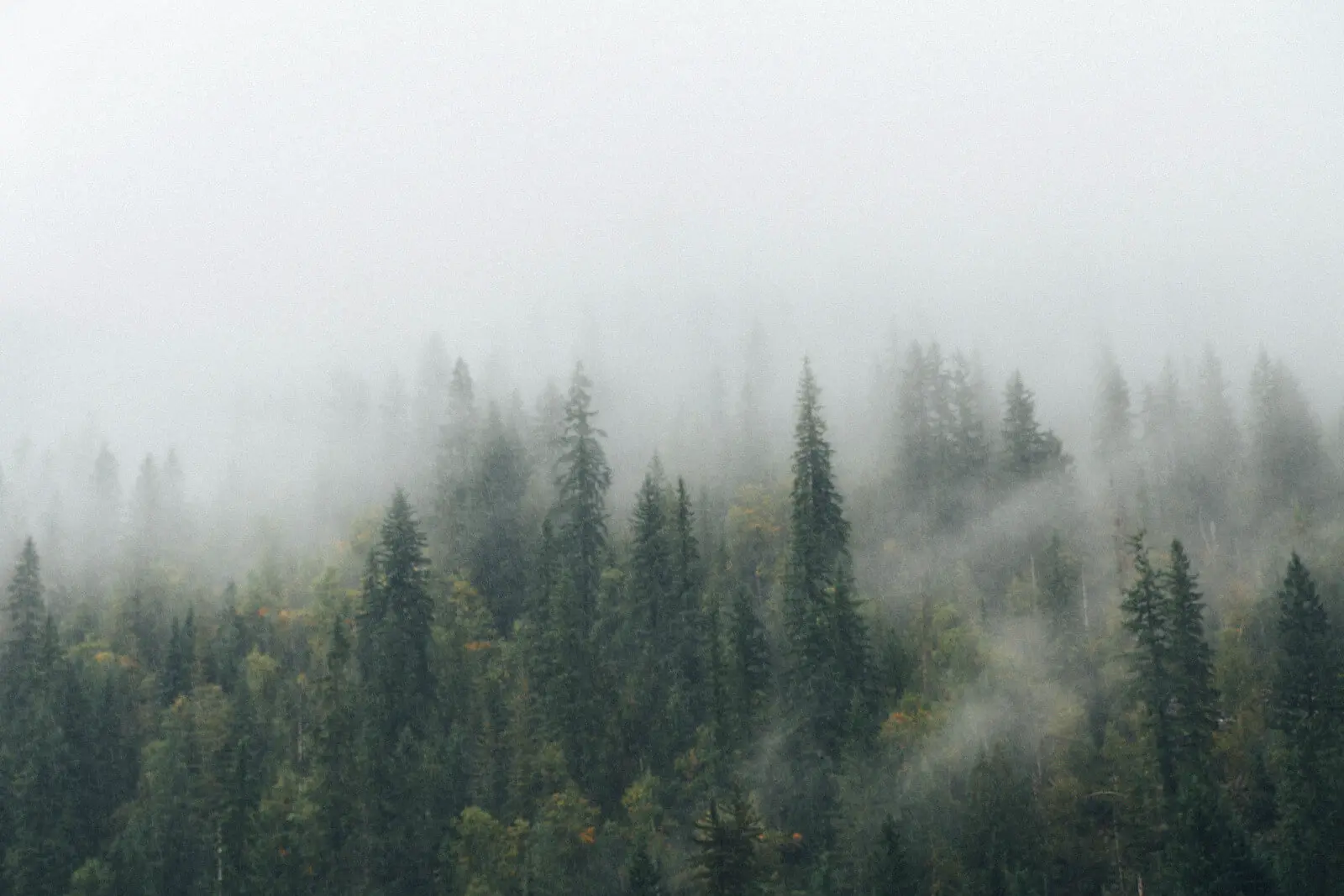
Explore Stefano Puliti's transformative forest research using YOLOv5 for drone-based detection and analytics in forestry.
Stefano Puliti is a researcher in forest remote sensing at the Norwegian Institute of Bioeconomy Research (NIBIO) in the national forest inventory department. NIBIO is one of the largest research institutes in Norway with approximately 700 employees. They contribute to food security and safety, sustainable resource management, innovation, and value creation through research and knowledge production.
In his research, drones and other proximal sensing techniques such as mobile laser scanning are used to produce advanced analytics required to answer modern information needs.
Much of the work he has been doing was done with the SmartForest project, which is a long-term research program financed by the Norwegian Research Council along with most of the forest industry actors in Norway. SmartForest’s objective is to improve the efficiency of the Norwegian forest sector by enabling a digital revolution transforming forest information, silviculture, forest operations, wood supply, and the overall digital information flow in the industry.
Stefano worked for many years with more traditional machine learning applications such as random forest or support vector machines. About three years ago, he realized that it was time to step up the game in the field of deep learning, which is still in the growing stage for the forest sector. Since realizing the tremendous benefits that researchers can draw from deep learning, Stefano has been developing several applications in the field of drone and machine-based vision.
"I have been using it since summer 2021, and have not abandoned it since! I have to say that, it was a “first-sight” type of love thanks to the ease of getting the YOLOv5 repo up and running. This was extremely valuable to me because at the time I was not very familiar with python and YOLOv5’s shallow learning curve was the deal breaker."
Stefano had resorted to object detection primarily to identify in drone imagery trees in poor health status either due to damage caused by abiotic (drought, wind, snow) or biotic agents (insects ad fungi). Since then, he and his colleagues have been developing a whole family of YOLOv5 detectors, from potholes to the road edge and whorl detectors.
"By the time I stumbled upon YOLOv5, I had unsuccessfully tried to train some object detectors in TensorFlow Object Detection API for some time. Then I ran into the YOLOv5 repo and (without much hope) attempted training a detector and with great surprise, I started the learning process with four lines of code. I am not sure one can define it as a thought process or rather a lucky strike but it started."
Most models are then deployed on their cloud solution (ForestSens) to deliver services to the forest sector. There, users can upload for example drone images which are then turned into actionable insights thanks to our YOLOv5 family of models. Some of their trained detectors are also deployed at the edge on forestry machines or on logging trucks.
Since the beginning, Stefano and his team had been developing a whole series of YOLOv5 models to be used to help in:
Further, they are also looking into extending their drone-based models for forest health and inventory to coarser resolution aerial and satellite image data.
With the latest YOLOv5 releases, they are very much looking forward to the image classification and semantic segmentation that YOLOv5 may offer. These capabilities will extend their ability to solve complex computer vision tasks in forested environments.
The ease of set-up both for training the models (docker version), as well as for the model deployment made YOLOv5 a great option for them.
“One of the aspects that always fascinated me about Ultralytics is the rather new business model that relies on open code at its core and offers pay products for non-specialists to access the power of deep learning. As a scientist, I appreciate very much Ultralytics’s openness and I find that it is a great way to accelerate the product’s development. As a result, YOLOv5 is seeing rather drastic upgrades in constant evolution thanks to the contribution of many data scientists and practitioners.”
When going to international conferences during the past year, I found that forestry researchers are either scared by the complexity of deep learning or do not think it can play a role in their research. In all of these cases, I suggested taking a half day of annotation and trying to train a YOLOv5 to understand its power and simplicity.
To put it in actual words I was actually saying: “Your method is not working? Then YOLO it!”
If you would like to stay up-to-date with Stefano Puliti and his work, feel free to follow his Twitter account.
Tag us with #YOLOvME on our social media with your very own YOLOv5 use case and we’ll promote your work to the ML community.